Ekip 2022 Workshop in Vienna
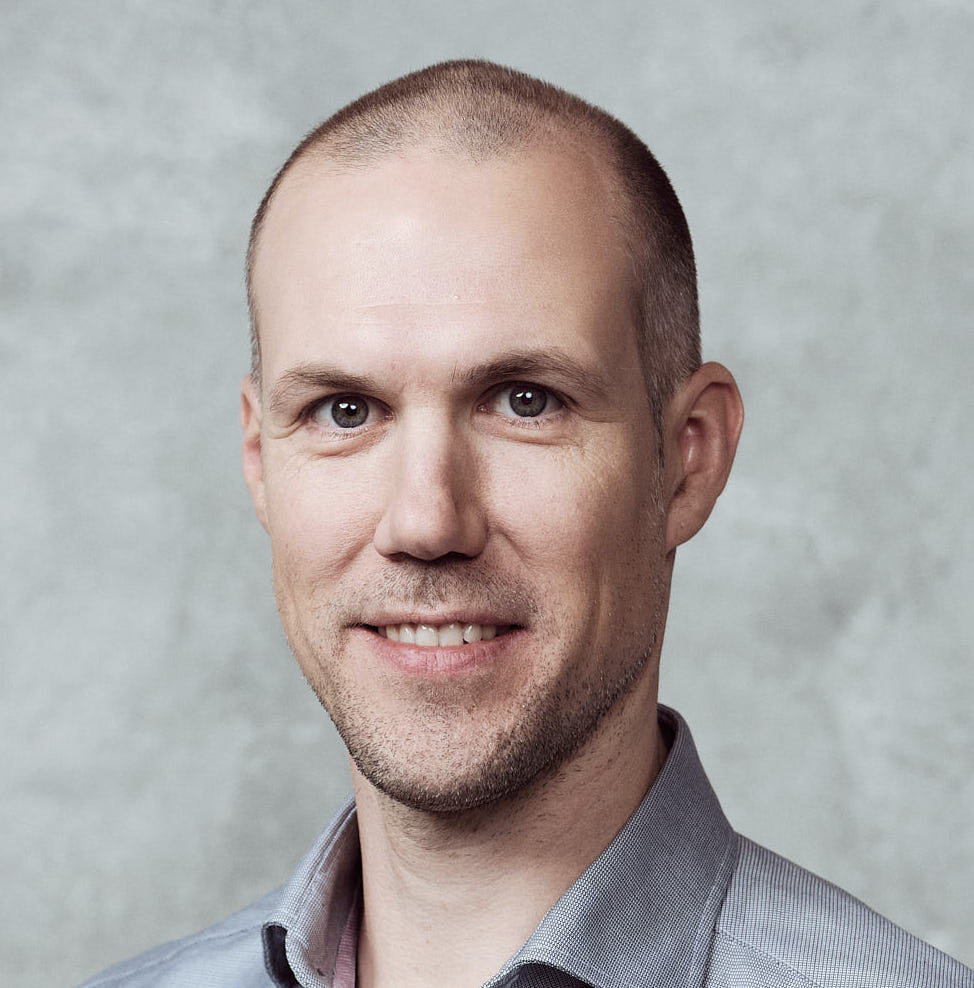
2022-03-25
How does ethical AI work practically? How do I develop AI systems that are trustworthy?
Exciting and important questions that are becoming urgent not only because of the ever more complex and powerful AI systems, but also, quite specifically, because of the approaching AI regulations.
These questions can only be answered by a diverse team from different disciplines. The task of developing trustworthy AI systems cannot be solved on a technical level alone.
We therefore held the first EKIP workshop. At the beginning of March, experts from the social sciences, philosophy and computer science met in Vienna to discuss how ethical AI can succeed in practice.
There are already many good suggestions of principles for ethical AI. However, how to implement these in concrete development projects is an open and varied question. In the workshop we presented a practical case study and applied our framework for trustworthy AI to it.
The case: in medical diagnostics, digital imaging processes are used to detect anomalies in the aorta. In order to arrive at a diagnosis, radiological examinations are usually discussed in the clinical process between the radiologist and the physician who is treating the patient. Computer-aided second opinions are already widely used today. In the near future, AI systems, especially those based on deep machine learning, will be able to use radiological images to come up with precise diagnostics with very high accuracy. How should such systems be used? Are they replacing one or more doctors? Is it at all ethically justifiable to use such systems (assuming they actually work that well)? And above all: how should such systems be so that they can be used with confidence? And what does that mean for their development?
In the workshop, we pursued these questions with regard to the concepts of explainability and responsibility.
If a radiologist or treating doctor takes over the diagnosis of an AI system, she would have to sign the report herself. In doing so, she effectively takes responsibility for the outcome of the AI system. But isn’t the responsibility actually distributed? And can and shouldn’t you only take responsibility for something that you fully understand?
So, does the AI system have to be fully explainable? Or should it be self-explanatory? And if so, how? What does explainability mean in this context?
An approximation: A trustworthy AI system should explain itself to anyone who asks (answerability). An explanation is sufficient if it satisfies and “feels good” (which in turn needs to be further defined).
An AI system that makes diagnoses based on medical images would therefore have to offer explanations for doctors, for patients, for specialists who check the algorithm and probably for many more. These people can only take responsibility for their part of the AI system if they have sufficient understanding of the explanation that is offered. How does the AI system come to offer these explanations? This explainability must be considered in the development and design process of the AI system. And from all these perspectives for which it will offer explanations later.
Following an “Ethical Requirement Engineering” approach, explainability requirements can be formulated for the system to be developed. These can aim at validating the system (on a technical and statistical level), or demand transparency for uncertainties and model outputs or, for example, have as their subject the presentation of reasons.
An exciting discussion among the participants revolved around how exactly this explainability can be achieved. Can machine learning models be explained at all? What epistemological basis can be used for explainability in this area? Who explains or who provides the explanations? And shouldn’t the term ‘intelligence’ be deleted (or replaced) from ‘AI’ from these points of view?
What is clear: all these questions must be discussed from beginning to end in the development process and the application of AI systems. In all stages of the so-called ML-Ops (Machine Learning Operations) cycle, the considerations of “Ethical Requirement Engineering” must be incorporated with the participation of philosophers, computer scientists, designers, psychologists, users, and patients.
Another important finding of the workshop: AI systems are never finished in their development and deployment. Rather, they should be understood as an ongoing service with continuous support. The problem to be solved with the help of the AI system requires constant checking, adjustment, explanation, and further development. Trustworthy AI is a service that consists primarily of dialogue between all those involved in the development and application of this AI. In this sense, we look forward to the next steps towards an applicable framework for Ethical AI development and a continuation of the dialogue within and beyond the EKIP team!
More on EKIP: https://ekip.ai