Synthetic Data – Potential Benefits and Limitations
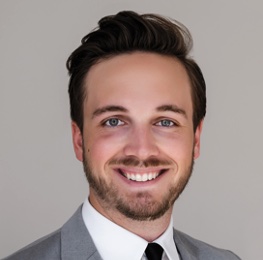
2020-03-04
In our last blog posts we discussed anonymization techniques under the GDPR and gave an introduction to Differential Privacy. Today, we would like to give an overview of synthetic data applications in AI, its potential benefits and limitations.
Due to the recent growth in Big Data volume, increasing interest in (and use of) predictive data analytics, and stricter enforcement of data privacy laws, privacy protection of each individual is becoming very important. Currently used anonymization techniques have shown to be cost intensive, error-prone and creating a false sense of security. One new approach trying to preserve all features and characteristics of the original dataset without disclosing any private information about an individual’s information in the data is the generation of synthetic data. Essentially, synthetic data tries to mimic real data.
Data Scientists can generate synthetic data from an original dataset with the goal of preserving all the important defining properties while at the same time no sensitive information can be deducted from the synthetic data set anymore. In its theory, the synthetic dataset can easily be shared and used for analytics and machine learning.
Example Use Cases & Benefits
Ideally, newly generated datasets contain no more sensitive information that can be linked to an individual. Thus, it should be safe to use for a variety of use cases in many (consumer-facing) industries.
- Cloud processing and migration (with synthetic data parties can process data to cloud infrastructures)
- Data sharing (synthetic data enables firms to easily share data internally or with external partners)
- Data analysis (analyzing synthetic data doesn’t fall under new GDPR regulations, which enables companies to perform big data analysis on the datasets, such as customer or medical patients data)
- Machine learning (accessing data to train machine learning algorithms is often a tedious process. With synthetic data external teams can gain access to other datasets quicker)
Trade-off between Privacy and Utility
Naturally, when working with completely synthetic datasets, the validity and completeness of the data needs to be evaluated. Research has shown that synthetic data generation does not always and completely preserve each individual’s privacy. Whatever technique is chosen to generate the synthetic data set, it is very important to disclose the underlying method and privacy guarantees – for example with Differential Privacy. Synthetic data hence is not a silver bullet; some companies claim they can provide best-in-class utility and privacy, but unfortunately is scientifically incorrect and marketing talk without substance.
Utility-wise, models try to look for trends and connections in the original data when generating synthetic data and may not completely preserve all properties and features desirably. In some cases, these issues will severely reduce the capability of the analyses and negatively affect the output.
Another factor is the quality of the original dataset. Since synthetic data is directly generated from an original dataset, its quality is also directly influenced by the quality of the original dataset. For example, adversarial perturbations may cause a model to misinterpret data and to then create inaccurate outputs.
Due to these limitations there is a need for verification. To receive validated and useful results the only way to guarantee an output to be accurate, the data user has to run the same analysis on the original dataset to make the results comparable, which in turn contradicts the whole purpose of synthetic data.
Disguise your valuable data with DQ0s synthetic data function
Because of this strong utility-privacy trade-off with synthetic data, we decided to include synthetic data only to a specific extent in DQ0. Since the external analyst has no direct access to the quarantined data and relies heavily on all the information the data owner provides, we integrated the feature of generating synthetic data for the data owner in a controllable, measurable way. So that the data scientist can use it for exploring and “getting-to-know” the data with a high level of privacy and good enough utility for these tasks.
Conclusion
Synthetic data is a great tool for testing and exploration and future research might improve the generation algorithms in a manner that validity and accuracy of the data becomes less of a problem or at least more transparent. As of today, synthetic data depends heavily on the original, underlying data and therefore either comes with the risk of disclosing private information or with very low actual utility.
Because of that we propose using DQ0 and its Differential Privacy mechanisms to keep your data as it is. This way, you gain the opportunity to really work with the original datasets and can trust the insights, results and privacy guarantees.
In future blog posts we will discuss synthetic data from a more scientific point of view.